A decade is a long time, about 1/8th of an average life span if you happen to live a long life. I came across Scott Alexander’s post where he wrote about his intellectual progress in 2010s and thought it’ll be a good idea to do the same for myself. When I had turned 30 two years back, I had looked back at the goals that the 20 year old me had. If you read that post, you’ll see that overall I feel that my 20s (and correspondingly, most of the 2010s) were very fulfilling. I started a company, fell in love and made myself financially independent.
This post is different as it’s solely about my intellectual progress. It’ll be hard to unpack an entire decade here, but let’s give it a shot.
Sabbaticals are highly underrated
Looking back, my entire decade’s intellectual progress only kick started 2 years ago. Running and scaling a company takes tremendous energy and after Wingify‘s founding in 2010, I didn’t get a good chunk of time to think about anything else. In fact, the trigger for my one month long sabbatical in December 2017 was a growing sense of intellectual decay. Startups make you psychologically strong and help sharpen your people skills but they don’t help you grow intellectually. (This is not to say people skills are easy or trivial – in fact, becoming a leader is incredibly hard).
I spent my sabbatical at home, doing only three things: thinking, reading and writing. The first thing I did was to start thinking about why Wingify succeeded while my previous startup attempts failed. That thinking led to this blog where I explored the theme of Inverted Passion, the idea that passion misleads entrepreneurs into building things that nobody wants and that the world rewards, not passion, but inverted passion. I’ve written many essays on startups ever since then and now feel that I understand the “laws” of startup success and that feels like intellectual progress to me.
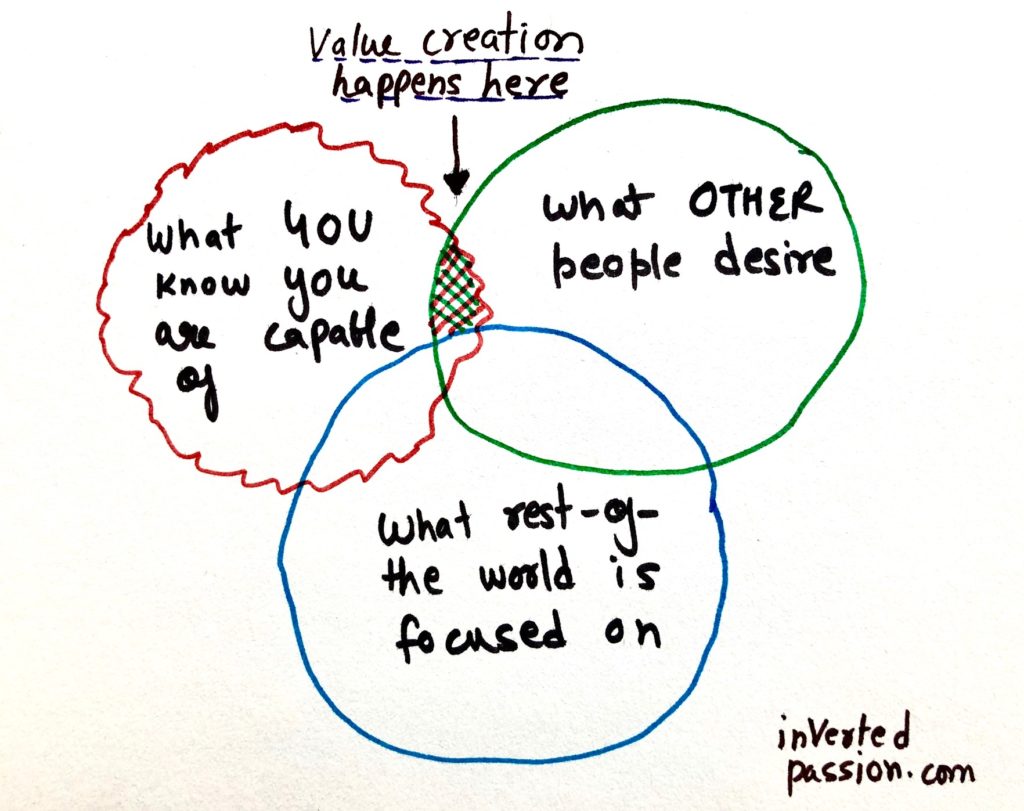
If I had not taken a sabbatical, I don’t think I would have thought deeply about what makes startups successful. So I highly recommend everyone taking a couple of weeks every year to simply think about the “laws” of their profession.
Rationality: v0.1, v1.0 and v2.0
I used to believe I was pretty rational but I was never rigorous about what rationality meant. How could I be rational if I didn’t know what it was supposed to be? Let’s call this level of rationality v0.1. At this level, whenever we’re solving a problem, whatever answer leaps to us from our intuition or gut, we make it our own and then we use our reasoning skills to justify that answer. Our brain makes us feel smart about finding an answer and we confuse it for being rational. Our brain does this because it’s job is not to find the truth but to help us (and itself) survive. And survival means – not necessarily finding the best or right answer – but finding a good enough answer that doesn’t piss off others. (Read my notes from the book Enigma of the Reason for more on this).
The next level of rationality – v1.0 – is when you realize that the map is not the territory and that thinking beyond initial hunches is actually pretty hard. I progressed to this level after reading an excellent book called Rationality: from AI to Zombies. If you want to be smarter, get familiar with the ideas in it. The key theme that it deals with is cognitive biases, how they lead to sloppy thinking and how you should train yourself to avoid them.
You’d think that once you’ve achieved v1.0 level of rationality, you’ve reached the peak. Now you can calculate everything with perfection and find all the right answers to all questions. If everyone in the world were rational, there will be no wars. At this level, rationality – as defined in v1.0 – seems like a panacea and an ideal goal for humanity.
But, if that’s the case, how you would you explain the fact that most people lead their lives not panicking that they might not be thinking perfectly, and yet live without drastic, major life altering, errors? Also, how would you explain the cruelties done by the so-called rationally designed USSR? Well, it’s because rationality is a very narrow way of dealing with the world. Evolution has programmed our intuitions to help us survive and in many real world situations, our thinking may not be sufficient to grasp the complexity. The next level of rationality – call it 2.0 or meta-rationality – is all about not harming yourself by being too smart. Our brains think in very linear, narrow fashion while the world we inhabit is interconnected with complex interactions.
For example, many atheists ridicule religion on the assumption that people are foolish enough to believe in the supernatural. But what if religion is simply a way for groups to bind with each other and rituals are a way to show allegiance to a group? The book Elephant in the Brain talks about it (read my notes). Rationality 2.0 is acknowledging the tacit knowledge we all have that cannot be described in words. It’s about getting rid of the arrogance of raw intellectualism and realizing that good engineers make terrible leaders. Making progress to this level, you realize the difference between normative theories (what people should do: utility maximization) and descriptive theories (what people actually do: survival).
My current understanding of rationality is that whatever stands the test of time is ultimately rational. (Some people call it the Lindy Effect.) If you’re too smart for yourself but end up with something you regret, then you’re not rational – you’re just arrogant. Whatever has stood the test of time (emotions, gut feeling, rituals) has knowledge that we will never fully understand, so it is wise to not throw them of the window.
Brain, Active Inference and Consciousness
I took two online courses on brain: one was Nancy Kanwisher’s course on Human Brain and the second one was Christof Koch’s course on Neural Correlates of Consciousness. Both helped me understand how neuroscientists study the brain and what they’ve found out about it. I realized that we’re still in very early phases of understanding brain but there are some very impressive results. I think the biggest insight for me was the compartmentalization of brain – for example, we have a dedicated region for recognizing faces and if such region is stimulated electrically, the patient starts seeing faces everywhere (even on toasters).
I understood a lot of details about how brain works but lacked an explanatory theory. Perhaps brain is the way it is because it evolved that way? In physics, seemingly disparate phenomena – planets, stars, galaxies – can be explained using the same principle. Is there a similar Newton’s theory of brain? One candidate theory – the free energy principle or it’s cousin predictive processing – comes close. This theory suggests that brains models the environment to anticipate and avoid potentially catastrophic environmental situations, and the better this modeling, the higher the survival rate. This theory suggests that the brain makes us seek food because it doesn’t contain a model of the world where it survived without food. This principle is also known as active inference because brain is making an inference about the environment (no food), assigns it a low probability of finding itself in such a state and actively works to seek a high probability environment (lots of food).
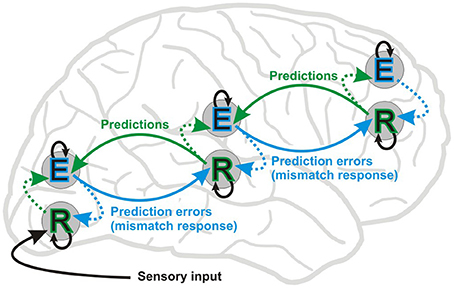
At the level I’ve written, the theory sounds very high level but that’s the beauty of it. This simple principle is supposed to explain many things – how life arose, how locomotion happens and even how brains are structured. The theory is still in active development but some parts of the theory is empirically supported. It’s exciting because it aligns so well with the idea that rationality = survival.
Proponents of active inference claim that it can explain consciousness too – they say that consciousness is nothing but a simulation of model of the environment that includes the agent itself. Even if this explanation turns out to be right, it’s merely explaining the easy problem of consciousness. What about the hard problem? How do you explain the redness of the red, the sweet smell of musk and the beauty of piano music? Besides free energy principles, there are a few more attempts to explain the easy problem (read Integrated Information Theory or Global Workspace Theory) but not many have dared to touch the hard problem. After scratching my head for long, I had to finally admit that perhaps some version of Panpsychism holds the key to explaining why red feels like red. Yep, accepting that everything – from electrons to quarks to rock over there – may be conscious is hard to digest but it seems the currently only plausible way to explain how our sensory qualia can arise out of material world . (Even though Panpsychism is an idea, there’s a resurgence of interest in related ideas with some even trying to put this theory into a physics-like framework).
Deep Learning, statistics, machine learning and probability
Before I started Wingify, in my college and school days, I used to love machine learning and AI. Before deep learning shook the world in 2010s, I used to code Neural Networks in Visual Basic 6.0 . In the last decade, neural networks made tremendous progress – they started becoming really good at translating languages, playing chess, captioning images, transcribing audio and a lot of other tasks that previously were thought out of reach for non-humans. Feeling left out, I caught up with deep learning via a course taught at fast.ai and got myself up to date with convolutional networks, recurrent networks and more.
After getting up to speed, I got my hands dirty by exploring several projects of my own. In one project, a neural network generated philosophy while another painted and yet another learned to beat me at Pong. I also built a network that captioned images. Simultaneously, I also thought hard about why deep learning is so effective and it occurred to me that it’s probably because our world is also deep and hierarchical.
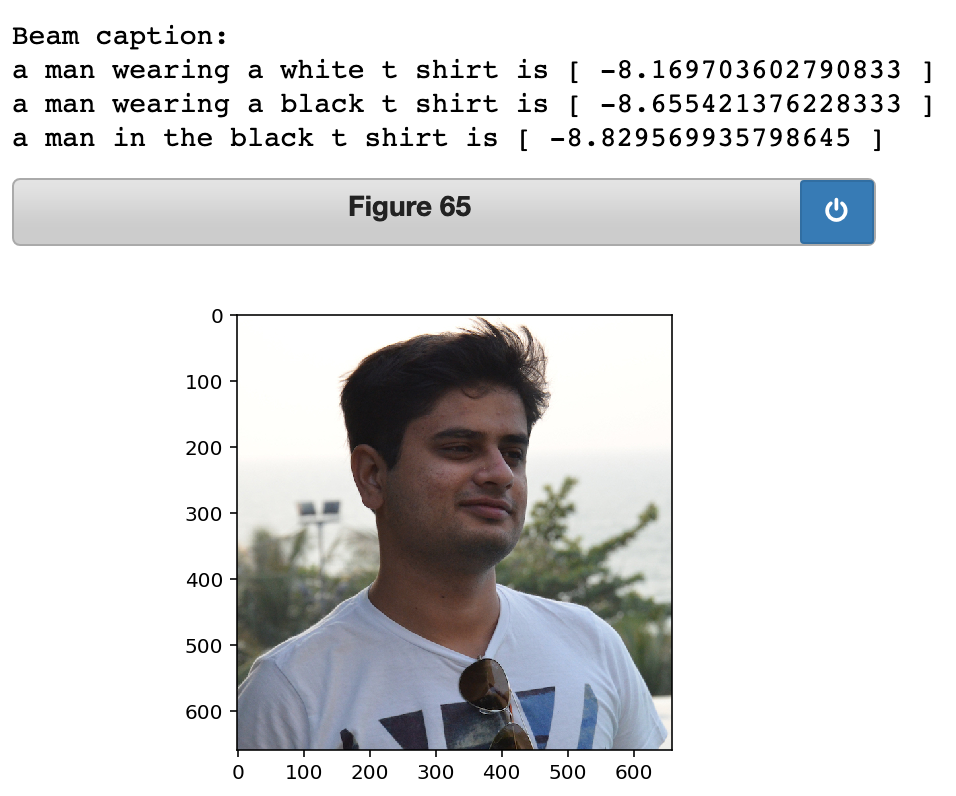
My most popular project was a Bayesian neural network. Thanks to books Statistical Rethinking and Bayesian methods for Hackers, I managed to get a solid intuition about what bayesian way of looking at the world is and that has led me to understand foundations of statistics and machine learning. For example, I now appreciate the difference between model and data, and understood how model can never be derived from the data but must be supplied a priori. In traditional (frequentist) methods, such models are implicit and what Bayesian approaches do is simply make all such assumptions about the generative model explicit.
Algorithmic complexity, complex systems and chaos theory
We loosely talk about complexity but it’d be good to rigorously define it. Thanks to another online course – Algorithm Information Dynamics – I understood the key idea that something is complex not because it looks big and complex but if it takes a lot (of information/code) to describe it. In other words, complex things are harder to compress. The digit pi can be expanded infinitely long but the code to generate it is small. Hence pi is simple. But, a string of truly randomly generated digits is hard to compress. Hence that set of digits is complex. This idea, in a rigorous form, goes by the name Kolmogorov complexity or Minimum Description Length.
The insight that complexity = length of code expanded my worldview significantly. Suddenly, Occam’s Razor made sense. If laws of physics are generated randomly, shorter/simpler laws are more likely to be generated than longer/complex laws. So, given everything else is equal, we should prefer simpler laws over more complex laws. Similarly, machine learning and intelligence started to look like compression to me. If an intelligent agent is able to deduce regularities in data, it can store such laws instead of raw data.
On a related note, I also ended up studying complex systems and chaos theory. While complex systems are the ones that look complex but behave in predictably simple ways, chaotic systems are the ones that look simple but behave in unpredictable ways. An example of complex system is a market economy where millions of interactions collectively produce a price which can be understood as equilibrium point of demand-supply curve.
Even though a complex system may have emergent properties that are simple to understand, our ability to predict them is limited. There are so many moving parts in a complex system that we can never isolate environment from a complex system, hence making physics or engineering style predictions impossible. Yet we keep finding economists and pundits making predictions about macro-economic indicators such as GDP growth. Go figure!
Chaotic systems are unpredictable even when we can perfectly isolate them. An example of chaotic system is a logistic map which starts out looking simple but becomes practically unpredictable. In fact, you can use the simple equation behind logistic map to generate seemingly infinite random numbers.
Physics – beyond pop-science
Throughout the 2010s, I’ve read many popular science books. But by the end of the decade, I started finding key ideas getting repeated. It gradually dawned on me that I read far too many pop-science books and it was perhaps time to graduate to the next level. This meant reading physics textbooks and for that I needed to brush up my math skills.
The excellent videos by 3Blue1Brown helped but without solving problems yourself, it always feels like you’ve understood something without really understanding it. It happened to me as well. I’d watch a linear algebra video. Smile, feel smart, nod and then move on. What actually helped me really (re)brush basic concepts was the book What is Mathematics. It explained the basics very simply and more importantly helped me get comfortable with mathematical notation.
Once I felt comfortable with calculus (which is what physics mostly relies on), I did an online course on statistical physics by Leonard Susskind. This course finally helped me understand what entropy really was and why it’s widely misunderstood.
Quantum Mechanics had always seemed like a mystery to me, so recently (but before the decade ended), I decided to read an actual textbook on it. I started with Quantum Mechanics: The Theoretical Minimum but quickly realized it depended on classical mechanics concepts (such as Lagrangian and Hamiltonian) that were never introduced to me before. So, I had to first read Classical Mechanics: The Theoretical Minimum.
Now, finally, I feel less intimidated by the woo-woo mysterious ideas such as “world is a hologram”, “electrons are both a wave and a particle” and “inside a black hole, space becomes time, and time becomes space”. I cannot yet derive results and theorems by myself but I have one level deeper understanding of reality as compared to pop-science books.
Financial bets, investments and why it’s so hard to beat the market
To me, investments had always been intellectually uninteresting and that’s why I had worked with finance and investment professionals to help manage my investments. However, watching how they decide, what they recommend and how they make up stories to justify their opinions got me interested in learning the subject myself.
I spent significant time studying how successful investors like Warren Buffet invest, took a tally of my past investments and tried analyzing what makes an investment good. From all this, it became clear that good returns come from good companies bought at a discount to their fair value. But, first, how do you estimate fair value of a stock? Second, even if you know the fair value for a stock, if everyone knows which ones are good companies, their price gets bid up beyond the fair value. And if a company stock seems to be available at a discount to fair value, the market has figured out that it’s probably not a good company and that your estimate for fair value is probably wrong.
Long story short: stock picking is hard but everyone believes they’ve got it right because they can cook up a story to justify their beliefs. But a rational investor should first face the fact that most expert stock pickers and mutual fund managers are not able to beat a dumb passive index (like Nifty in India or S&P500 in US). If not stock picking, how should one invest? The question led me to think about investments from first principles. And as index funds emerged as a clear winner, this led me to think about why indexes beat even the likes of Warren Buffet.
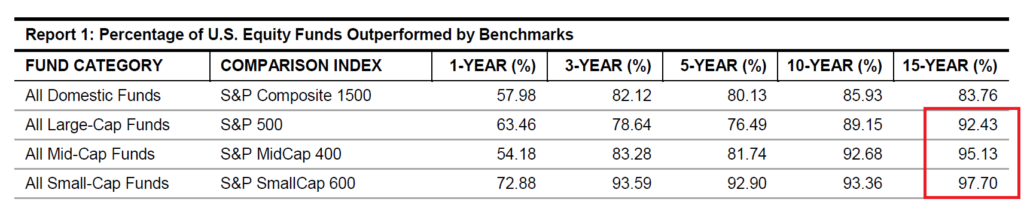
In the last one year, I got familiar with many terms that investment professionals use: Sharpe Ratio, Modern Portfolio Theory, Efficient Frontier, CAPM and Factor Investing. This led me to the wonderful world of Quants, high frequency trading and an increasing application of machine learning in finance. My current take on the investments is that it’s easy to produce sophisticated theory, and easy to over fit that theory to historical data. Once you have an overfit theory, it’s also to produce good narratives why that theory is correct because humans or markets behave in a certain way.
This science-like process gives false confidence and often the very same theories that look good on paper, fail spectacularly when used in live. This happens because markets are not like our universe that ticks along with predictable laws. Markets are made up of people and how they behave is mostly unpredictable because whatever predictability is there quickly gets exploited for profit. Losers exit the market and what remains is unpredictability that is hard to exploit. Having said that, I feel that markets are not entirely unpredictable. Both on macro and micro level, predictable human behavior and concerns about risk / anticipation of reward creates constantly-shifting opportunities to get an edge over the guaranteed, risk-free return provided by the central banks. So even though there are no eternal laws in finance, local opportunities arise regularly for a determined investor.
Evolution, Limits of understanding, Truth, Language, Philosophy and Science
Learning inevitably makes you greedy. You want to be able to explain everything with certainty. It’s natural to ask if a theory of everything is possible, and once it’s discovered, will we settle all debates for good?
Well, it’s becoming clearer to me that what we understand is not reality itself but models of reality. Our brains evolved to help us survive and not to understand reality. This is why it seems counter-intuitive that an electron is best described not by a billiard-ball like point but a cloud of possibilities. What does a cloud of possibilities even mean? I have no idea but I sure can write an equation for it!
As we gain deeper understanding of reality, we get further removed from our intuitions and language becomes ever-more blunt in describe what really is going on. This holds true not just for electrons and black holes but for complex macro-phenomena such as biosphere and social networks. Does any single person really understand how Trump got elected as the US president or what happened to Dinosaurs? Sure, we have our theories but they seldom exhaust the objects we put under their scrutiny. This is the position taken by an exciting new philosophical movement: Object Oriented Ontology. This philosophy puts all objects – things, events, relationships, humans – on an equal footing and suggests that while we can understand certain aspects of objects, they can never be fully understood. We can think of hammer as a tool for us, but it’s also apparently a place for dust to settle and an infinitely more things that will never occur to us.
That there are things that we will never understand is not just hand-wavy. Right from Turing’s halting problem to NP-complete problems, there are proofs that certain questions are either impossible to answer or impossible to answer within universe’s lifetime. You truly cannot understand everything that is out there.
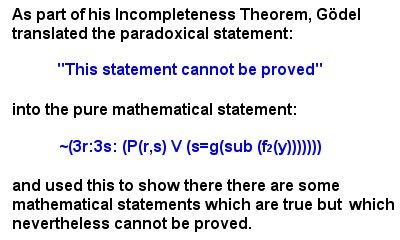
Couple our inability to understand reality as it is with the fact that when scientific paradigms change, even our own interpretation of it changes. Before Einstein came along, time seemed to tick at a constant pace across the universe. Before quantum mechanics, electrons were thought to be little billiard balls. Throughout history, reality did seem to be what scientists believed it to be. There was no reason to suspect that time was anything other than a constantly ticking universal clock. But then scientific revolutions always change whatever we thought to know for sure. How does then one believe with certainty in currently accepted ideas such as the Big Bang or dark matter?
Perhaps a pragmatic view of science is to not literally believe that the universe was created 14 billion years ago but that this number is simply a data point that came out from our currently best equations and that it can further be used to predict other numbers. I’m not suggesting that scientific facts are not useful. Like good machine learning models, they help us make predictions. I’m simply suggesting that we should perhaps confuse our deduced scientific models as what the reality actually is. Remember that there are always multiple ways to describe observations/data. Our description evolves over time but reality stays the same.
What the hell is then reality? Our current scientific and philosophical worldview is shaped through the western thought that started with the Greeks and continues to be dominant mode of thinking worldwide. They thought they can understand the universe through their intellect and actually got pretty far with it. (Look where the western science is today). This attitude also reflects in the western ideal of progress. We, the humans, are expected to continuously make progress on all fronts – including our understanding of reality. But what if that attitude is misguided, and that there’s no such thing as the arc of history? What if progress is not guaranteed and that actual reality will forever be closed to us? It seems that’s what’s turning out to be as quantum mechanics and black holes continue to make a mockery of our intuitions. It seems we’ve hit an interpretative roadblock. In contrast to the western philosophy, when I read about Hindu philosophy it seemed that perhaps Hindu sages were right when they said the unknowability of reality is fact that needs to be celebrated, not contested.
Now, how I’m using the word reality may be very different than how you use the word. So let me be clear. When I say reality, I mean the raw structure of our world and, more importantly, its raison d’etre. It’s okay if you mean something else by reality. Most disagreements happen but people use same words in different senses all the time (without making that explicit). Because of this philosophy ends up a game of persuasion. Questions like whether free will exists may have different answers, depending on the context. Also, because of this ambiguity, meaning of life is unthinkable. Same goes for the word truth. Things represented by the same words or concepts might be true or false, depending on what slice of context you subject them to.
My current attitude towards science is that it’s better to say which theory is more useful in which context, rather than saying what’s true. For example, motion of planets can be described by both Newton’s laws and Einstein’s laws. It’s silly to say Einstein’s laws are truer than Newton’s laws. There’s no such thing as truer. Truth is absolute, and hence it’s best to avoid it.
The 2020s
So that was all I learned in 2010s. I’m sure I have missed a few things, but I’m confident that it covers all the major themes.
What am I excited to learn in the current decade of 2020s? I’d like to keep myself open to serendipity but I have a few broad topics I’d like to go deeper into. The list includes physics, evolution, machine learning, consciousness research, and complex systems.
I usually share whatever I’m learning on my Twitter. My learning journey resulted in these 100+ twitter threads. So if you want to learn along with me, consider following me on Twitter.
Thanks Aakanksha Gaur (@sia_steel) for proof-reading the post.
Join 200k followers
Follow @paraschopra