Not winning does not imply losing.
(a thread on perils of binary thinking)
1/ We recently ran an A/B test and here were the results.
In the test results we still don’t have 95% statistical confidence (probability to be the best) but we’re going ahead and implementing the variation on homepage.
Why?
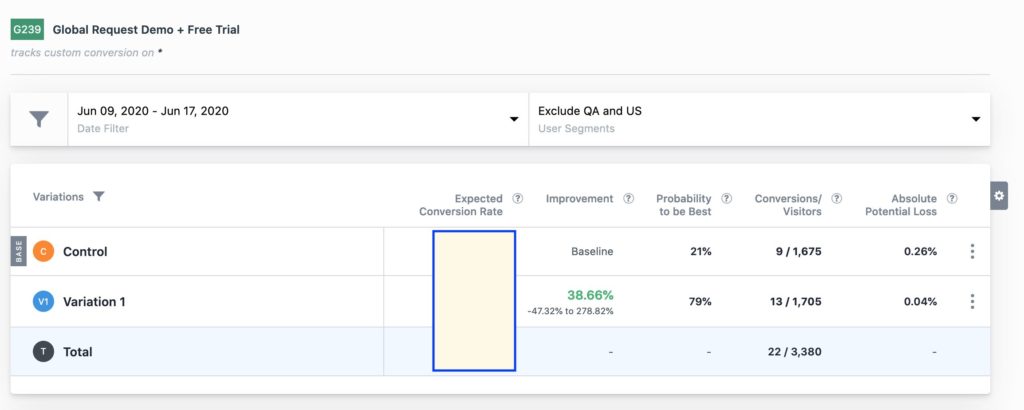
2/ Because, and here’s the key idea.
Not getting a winner is different than having a loser!
Allow me to unpack..
3/ Whenever we talk about test results, our immediate hunch is to jump and categorize into binary categories: either something is a winner or a loser.
But in real world, there are no binary categories.
4/ There’s no magic threshold at which a variation suddenly becomes a winner.
The 95% confidence threshold is arbitrary and whether you act based on it (or some other threshold) depends on your goals.
5/ So, even though the variation may not be good, we know that it’s likely that it’s not bad.
Rather than getting trapped into discussions about winner/loser, what matters is what is likely impact of implementing this on website.
6/ In best case, variation actually turns out to be good.
In the worst case, it is similar to existing one.
(In the extreme worst case, it can be a catastrophe but if you see the range of uplift, it is much more likely to be better than worse)
7/ With data, it’s easy to fall into the trap of binary decisions about data while what matters is likely impact.
KNOW the difference between probability and impact.
Read first chapter of this book.
8/ TLDR: there’s negligible probability that that we’d have a catastrophic super-solarflare that will fry up our world’s electronics and internet.
But if that happens, it’ll be really, really bad.
9/ That’s it!
Hope you liked this mini-thread.
The take-home message is to not get fooled by probabilities by always keeping focus on consequences of probabilities.
This essay is a lightly-edited version of a Twitter thread I posted.
Join 200k followers
Follow @paraschopra