Just finished this book on the history of Bayes theorem and I highly recommend it.
In case you’re wondering what is it, keep reading.
(A thread on Bayes theorem)
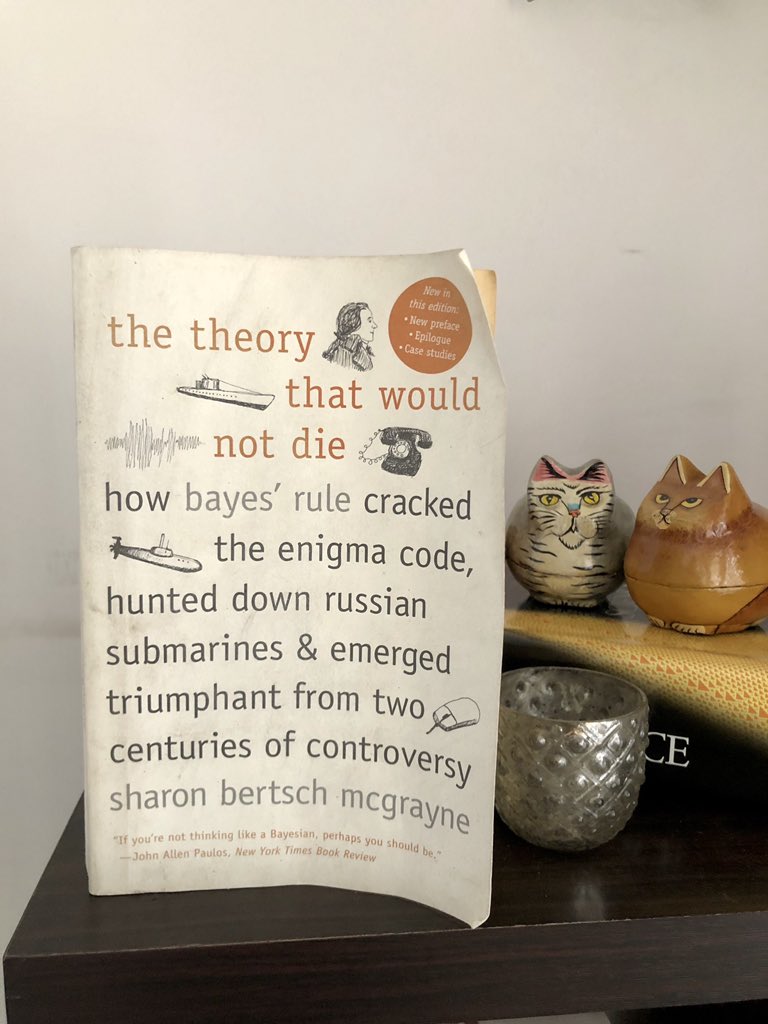
1/ Statistics is all about calculating probabilities, and there are two camps who interpret probability differently.
Frequentists = frequency of events over multiple trials
Bayesians = subjective belief of the outcome of events
2/ This philosophical divide informs what these two camps usually bother with.
Frequentists = probability of data, given a model (of how data could have been generated)
Bayesians = probability of model, given the data
3/ Most often we care about the latter question.
E.g we want to know given that the mammography test is positive, what is the probability of having breast cancer.
And not given breast cancer, the probability of test being positive.
4/ These two questions sound similar but have different answers.
E.g. imagine that 80% of mammograms detect breast cancer when it’s there and ~90% come out as negative when it’s not there (which means for 10% times it comes as positive even if it’s not there).
Then if only 1% population has breast cancer, the probability of having it given a positive test is 7.4%.
5/ Read that again: 80% times the mammography test works and yet if you get a positive, your chances of having breast cancer are only 7.8%
How is it possible?
Read this simple explanation.
6/ The math is simple:
- Chances that the test is positive when a patient has breast cancer = chances of detecting breast cancer when a patient has it * chances of having breast cancer in the first place = 80% * 1% = 0.8%
- Chances that test is positive when a patient does NOT have breast cancer = chances of detecting breast cancer when a patient DOESN’T have it * chances of NOT having breast cancer in the first place = 10% * 99% = 9.9%
Now, the chances of having breast cancer on a positive mammogram are simply: % times you get a positive mammogram if you have breast cancer / % times you can get a positive mammogram. We calculated these numbers above, so this becomes 0.8%/(0.8%+9.9%) = 7.4%.
Voila! So even if a test works 80% of the times, it may not be very useful (if the population incidence rate is low, which is 1% in this case). This is why doctors recommend taking multiple tests, even after a positive detection.
7/ When you understand Bayes theorem, you realize that it is nothing but arithmetic.
It’s perhaps the simplest but most powerful framework I know.
Here’s another intuitive explanation of Bayes theorem (which also contains the breast cancer example we talked about) by @obonilla
8/ The key idea behind being a Bayesian is that *everything* has a probability.
So instead of thinking in certainties (yes/no), you start thinking in chances and odds.
9/ Today, Bayes theorem is behind many of the apps we use daily because it helps answer questions like:
– Given an e-mail, what’s the probability of it being spam?
– Given an ad, what’s the probability of it being clicked?
– Given the DNA, is the accused the culprit?
– And, of course, given the data, is variation better than the control in an A/B test? (FYI – we use Bayesian statistics in VWO)
10/ If you’re interested in getting into the rabbit hole, go through this self-study interactive guide recommended by @ESYudkowsky
11/ That’s it!
Hope you also fall in love with the Bayesian way of looking at the world.
If you have any questions, let me know. Happy to answer!
This essay is a lightly-edited version of a Twitter thread I posted.
Join 150k+ followers
Follow @paraschopra